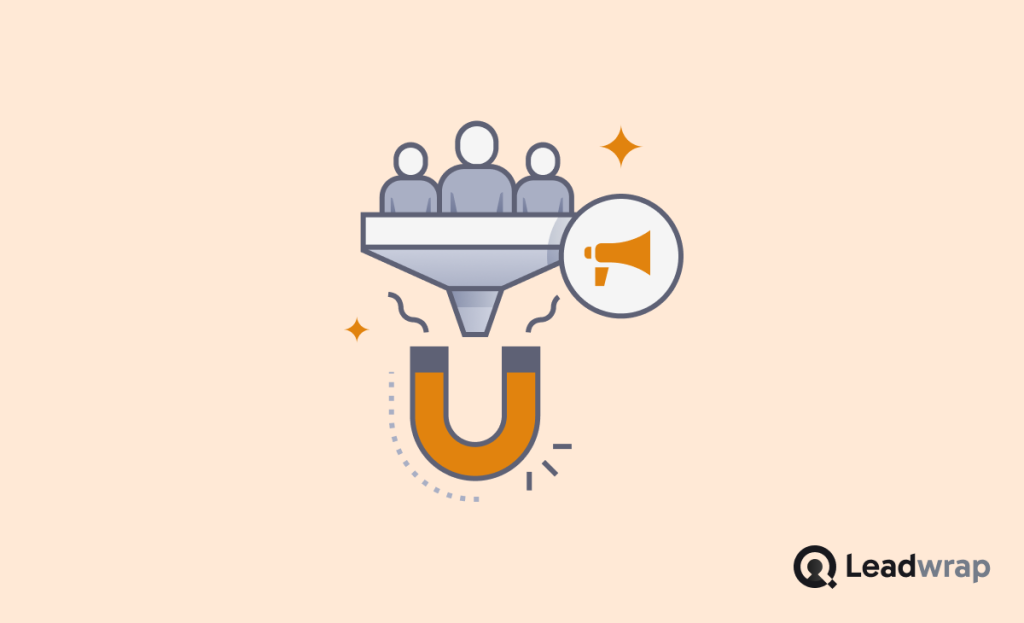
It has never been easier to find the high quality of leads. The old methods frustrate oneself on low quality prospects, overflowing and creating a vicious circle of frustration and suboptimal ROI.
Precisely, it is tough for businesses to turn leads to customers if they do not target customers properly. So the end result was missed revenue, wasted marketing and declining growth.
AI revolutionizes lead generation by examining behaviors, predicting intent and crafting personal outreach. That correctly serves the right business at the right time converting cold leads to wildly loyal customers.
How AI Changing the Future of Lead Generation
AI has been changing the lead generation from running, to well-oiled data led and personalized pipelines. With the help of advanced automation and predictive analytics, businesses are now capable to not just identify leads but engage them, convert with utmost precision.
i. Data-driven prospect identification
AI identifies lead quality with a lot of precision by using massive datasets. It uses to identify the hot prospects based on online activity, purchase history and behaviors patterns. So it takes the guesswork out and makes sure marketing actually get in front of the right people.
ii. Intelligent lead scoring
There are different lead quality machine learning models and they evaluate the factors simultaneously. – They focus activities, email interactions and past purchases that assist businesses in qualifying the right opportunities so sales teams can work on hot leads. Therefore Sales teams can get leads that are highest probability to convert.
iii. Dynamic personalization strategies
AI promotes tailored experiences that dynamically recalibrate content in real-time. Utilising user behavior over all channels, it also tailors email campaigns, website behavior and ad recommendations. The higher touch points have the ability to strengthen client relationships and drive conversions.
iv. Automated outreach and follow-ups
Conversational AI (chatbots and virtual assistants) enable automation of conversations. These are the tools that perform first interaction, field questions and subsequently automate the lead nurturing follow-ups. This decreases the turnaround time and also engages prospects without human interaction.
v. Predictive insights for sales optimization
AI examines historical trends and market shifts to forecast future sales. Businesses can anticipate demand, refine their strategies, and allocate resources efficiently. This predictive approach minimizes risks and maximizes revenue potential.
How Does AI Differentiate Between High-Quality and Low-Quality Leads
AI-powered lead qualification goes beyond surface-level metrics, using advanced algorithms to analyze data patterns and predict conversion potential. By leveraging machine learning, businesses can separate promising prospects from low-value inquiries, ensuring that sales teams focus on the most valuable leads.
Decoding buyer behavior through engagement patterns
AI meticulously tracks and evaluates a lead’s digital footprint—every website visit, email open, and social media interaction tells a story. High-quality leads frequently engage with content, revisit key pages, and interact with marketing materials, while low-quality leads display sporadic or minimal activity.
Harnessing predictive analytics for smarter lead scoring
By analyzing historical conversion trends, AI assigns predictive scores to leads, identifying which prospects share characteristics with past successful customers. This scoring system enables businesses to prioritize leads with the highest likelihood of closing.
Understanding intent through conversational AI
Natural language processing (NLP) deciphers the depth of interest behind customer inquiries, whether through chatbot conversations, email responses, or direct search queries. AI can distinguish between casual browsers and those showing strong purchase intent, ensuring sales efforts are directed toward genuinely interested prospects.
Mapping demographics to the ideal customer profile
AI cross-references a lead’s job title, industry, company size, and geographic location against an ideal customer profile (ICP). If a lead aligns with high-value customer traits, they receive a higher qualification score, while mismatches indicate lower potential.
Evaluating lead sources for trustworthiness
Not all lead sources are created equal. AI assesses whether a lead originated from an organic search, referral, targeted campaign, or cold outreach. Leads from high-value sources, such as direct website visits and referrals, typically indicate stronger buying intent compared to low-engagement channels.
How Does AI Handle Data Privacy and Compliance in Lead Generation?
Ensuring data privacy in AI-driven lead generation is essential for maintaining trust and complying with global regulations. According to a PwC report, 85% of consumers say they won’t do business with a company if they have concerns about its security practices.
i. Secure data collection practices
AI-driven systems collect data through encrypted channels and user-consented interactions. They rely on privacy-first frameworks to limit exposure to unauthorized access. This ensures that businesses gather leads while adhering to strict security standards.
ii. Compliance with global regulations
AI tools are programmed to align with GDPR, CCPA, and other data protection laws. They automate compliance checks, flag risky data usage, and enforce privacy policies. This helps businesses navigate legal complexities while protecting user rights.
iii. AI-powered anonymization
To minimize data privacy risks, AI anonymizes personally identifiable information (PII). By applying tokenization and encryption techniques, it allows businesses to analyze trends without exposing sensitive details. This reduces the likelihood of data misuse.
iv. Transparent consent management
AI enhances consent management by keeping detailed records of user permissions. It ensures that leads opt in before their data is processed, reducing regulatory risks. Automated tracking also enables businesses to update compliance measures when laws change.
v. Continuous security monitoring
AI-driven cybersecurity solutions detect potential breaches before they happen. According to IBM, AI-based security reduces data breach costs by 80%. These tools monitor suspicious activities, restrict unauthorized access, and strengthen lead data protection.
What Are The Main Challenges Businesses Face When Integrating AI into Lead Generation?
Integrating AI into lead generation offers immense potential, but businesses often face significant hurdles. From data quality issues to compliance concerns, overcoming these challenges requires strategic planning and the right tools.
Data quality and management
Poor data quality is one of the biggest barriers to effective AI-driven lead generation. A report by IBM found that bad data costs U.S. businesses $3.1 trillion annually due to inefficiencies and lost opportunities. AI models rely on accurate, updated, and well-structured data to generate valuable leads.
For example, if an e-commerce company uses AI to predict high-intent buyers but feeds it outdated or incorrect customer information, the AI may target inactive users or irrelevant prospects. Businesses must invest in data cleansing tools, such as OpenRefine or Talend, and establish rigorous data validation processes.
High implementation costs
Deploying AI for lead generation often requires significant investment in software, infrastructure, and AI talent. According to Deloitte, 53% of businesses cite high costs as a major barrier to AI adoption. AI-powered CRMs like HubSpot AI or Salesforce Einstein can cost thousands of dollars annually, making them less accessible to small businesses.
A case study from Airbnb shows that the company spent millions developing AI algorithms to optimize customer targeting. While it improved lead conversions, smaller businesses may struggle to justify such costs. To mitigate expenses, companies can start with budget-friendly AI tools like ChatGPT for lead qualification or Zia by Zoho CRM.
Complexity of AI models
AI lead generation tools, such as predictive analytics or machine learning-based lead scoring, can be complex to set up and fine-tune. A McKinsey study revealed that only 20% of companies successfully scale AI initiatives, mainly due to technical challenges.
For example, if a business misconfigures an AI lead-scoring system, it might rank unqualified leads higher than genuine prospects. Companies can address this by using pre-built AI solutions like Drift AI for conversational marketing or hiring AI consultants to assist with setup.
Privacy and compliance issues
AI-driven lead generation often involves gathering vast amounts of user data, raising privacy concerns. A 2023 Cisco Data Privacy Benchmark Study found that 92% of consumers demand transparency on how their data is used. Regulations like GDPR (Europe) and CCPA (California) impose heavy fines for mishandling personal information.
For example, in 2021, Amazon was fined €746 million under GDPR for failing to meet data privacy requirements. To stay compliant, businesses should implement AI-driven data anonymization tools and ensure AI models adhere to ethical guidelines. Platforms like OneTrust help businesses maintain compliance with global regulations.
Resistance to change
AI adoption often faces internal resistance, especially from sales teams worried about job displacement. A PwC survey found that 67% of employees fear AI will replace human jobs, causing reluctance to embrace AI-driven lead generation.
For example, when IBM Watson AI was introduced into sales operations, some employees initially resisted due to concerns about automation replacing traditional sales roles. Companies can overcome this by training employees on how AI enhances rather than replaces their work. Tools like LinkedIn Learning’s AI for Business Courses can help teams build AI proficiency.
Integration with existing systems
Many businesses use legacy CRMs or marketing automation platforms that do not seamlessly integrate with modern AI tools.
For example, a real estate firm using an outdated CRM may struggle to integrate AI-powered chatbots for lead qualification. To bridge the gap, businesses can use middleware solutions like Zapier or MuleSoft to connect AI tools with existing software.
Final Thoughts
Artificial Intelligence is reshaping the lead generation muscle by making it smarter, quicker and much much more efficient. Using advanced data analytics, predictive analytics with automation for the right audience targeting businesses leverages these to reduce dead efforts and yield higher conversions. AI chatbots, custom marketing and lead scoring make sure leads are engaged by sending the right message at the right time.
Keep your eyes peeled — The more AI evolves, the greater will be its relevance in lead generation. Based on AI-driven companies will win the competition and magnifies revenue while boosting client experiences. Tomorrow belongs to those who love AI and unleash data into actionable insights for exponential business growth.